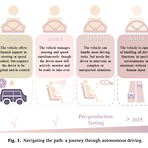
Introduction History 1970년대 영국 도로 연구소에서 자율주행 비디오 공개로 관심 얻음. 1980년대 다양한 대학들이 자율주행 연구 참여 2010년대 초, 차량 제조업체들이 참여 시작 Levels of Driving Automation 현재 수년동안 Level 2 에 머물고 있음 딥러닝의 발전으로 한계를 넘고 있음 딥러닝의 단점으로 문제도 있음 : the blackbox and inexplicable nature of neural networks Research Trend 현재 분야 내 중요한 키워드들을 시각화 한 모습 중요한 Sub part Perception and Loacalization Motion Planning and Decision-making Simulators and S..
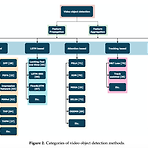
2021.11.30 Review https://pdfs.semanticscholar.org/87db/49a14a1dd0e3d672e1144dc13354d892558d.pdf https://www.semanticscholar.org/paper/A-Review-of-Video-Object-Detection%3A-Datasets%2C-and-Zhu-Wei/3c03cb37863eea4be5e01f407d6899620dc4d254?p2df www.semanticscholar.org --- Shortcomings of frame by frame basis object detection in video lack of computational efficiency due to redundancy across image ..
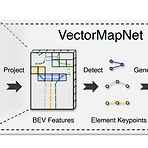
과거 리뷰한 내용을 업로드 합니다. --- 1.Abstract 자율 주행 시스템은 HD 시멘틱 맵 요소를 사용한다. 존재하는 방식들은 시멘틱 맵 문제를 메뉴얼적인 annotation으로 풀지만 확장성 문제가 있다. 최근의 방식들은 dense한 rasterized segmentation 예측으로 맵을 구성하지만 개개인의 맵 요소의 인스턴스 정보를 포함하지 않거나 vectorized map을 얻기 위해 휴리스틱한 post processing을 요구한다. 이러한 문제를 해결하기 위해 end-to-end로 Vector화 된 맵을 얻을 수 있는 VectorMapNet을 소개한다. VectorMapNet은 onboard sensor observation을 갖고 birds-eye view의 sparse한 polyli..
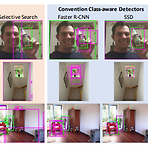
https://arxiv.org/pdf/2011.14204.pdf --- Proposed by Amazon Alexa Natural Understanding Team Limitations of conventional class-aware detection Hinders the adoption of trained detectors in real-world applications due to the added cost of retraining them for application specific object types such detectors cannot be used in applications like obstacle detection, where it is more important to determ..
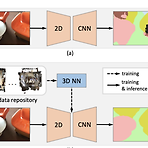
2022.02.08 Review --- https://arxiv.org/pdf/2104.02243v2.pdf Background Semantic Segmenation classifying each pixel in an image from a predefined set of classes Knowledge Distillation Process of transferring knowledge from model to other model that has different structure usaually, model compression method in which a small model is trained to mimic a pre-trained, larger model start to research f..
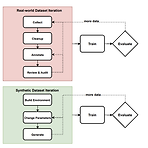
2022.04.05 Review --- https://github.com/MCG-NJU/AdaMixer GitHub - MCG-NJU/AdaMixer: [CVPR 2022 Oral] AdaMixer: A Fast-Converging Query-Based Object Detector [CVPR 2022 Oral] AdaMixer: A Fast-Converging Query-Based Object Detector - GitHub - MCG-NJU/AdaMixer: [CVPR 2022 Oral] AdaMixer: A Fast-Converging Query-Based Object Detector github.com https://arxiv.org/pdf/2203.16507.pdf --- Goal To simpl..
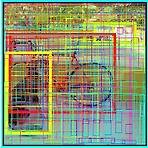
2022.10.13에 직접 리뷰 했던 것을 좀 지나서 블로그에 올립니다. 최신 정보는 아닌 점 참고 바랍니다. YOLO (You Only Look Once) Single stage real-time object detector Performance, Accruate, Active community featurized through a backbone, combined and mixed in the neck, passed along to the head YOLO-v7 AlexeyAB, WongKinYiu AlexeyAB took up the YOLO torch from the original author. Joseph Redmon, when Redmon quit the CV industry due to e..
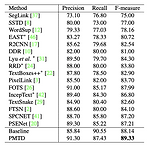
textbox++ 이걸로 트레이닝 해서 베이스라인으로 해봐도 괜찮을것 다른 detector 모델로 바꿔도 EfficientNet 리딩 해주심 다른 페이퍼 두개 읽어보기 중요 백본이뭔지 디텍션알고리즘 - 1stage - yolo 2stage - rcnn 우리는 1 stage (요즘 많이 쓰임) OCR -> 다른 거 뭐 쓰는지 확인 textbox는 인풋크기가 크기 때문에 작은것까지 뽑을 수 있지만, 테스트 사진에서 다 찾으면 좋고 적어도 두번째 C04까지 Vertical 구역번호를 Classification 을 활용 3/27 Idea : 사실상 텍스트플레이트가 아래쪽으로 내려올 일이 없을 것 같은데 그걸 고려하면 어떨까? (사진을 잘라서 쓴다던지) ICDAR 2015 텍스트 검출 - opencv contou..
VITON - Refinetwork 분석 3.3. Refinement Network VITON의 정제 네트워크 GR은 거친 흐린 영역이 변형 된 대상 품목의 현실적인 세부 사항을 활용하도록 훈련됩니다. 뒤틀린 의류 품목. 우리는 거친 샘플의 생성 된 영역의 세부 사항을 채우기 위해 대상 의류 이미지 C에서 직접 정보를 빌립니다. 그러나 제품 이미지를 직접 붙여 넣는 것은 사람의 자세와 몸 모양에 따라 변형 된 옷의 변형으로는 적합하지 않습니다. 따라서 그림 4에서와 같이 shape context matching [3]을 가진 thin plate spline (TPS) 변환을 추정하여 의류 항목을 왜곡한다.보다 구체적으로, 우리는 c의 전경 마스크를 추출하고 모양 컨텍스트 TPS warps [3] 이 마스..